Quality control systems based on deep neural networks
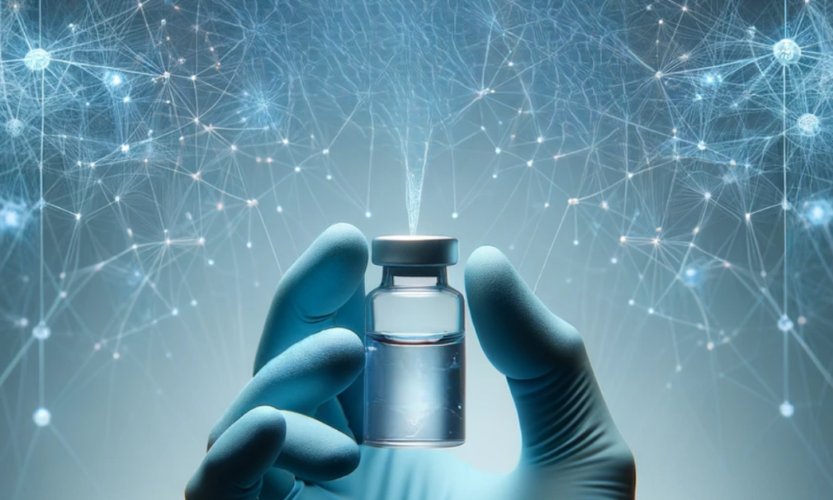
Modern quality control has more and more sophisticated tools at its disposal, such as vision systems based on the so-called deep neural networks which contribute to much higher precision and reliability of automated production control and facilitate the process of adapting the system to new formats or products. What is the superiority of vision-based quality control systems over traditional ones?
The more accurate the analysis and the faster the reaction to the appearance and detection of defective packaging, the lower the potential losses in manufacturing.
To put it simply, the machine vision which forms the basis of automated quality control on high-speed production lines is a vision system (camera system) processing data about the object and eliminating defective products that do not match the pattern.
Modern quality control has more and more sophisticated tools at its disposal, such as vision systems based on the so-called deep neural networks which contribute to much higher precision and reliability of automated production control and facilitate the process of adapting the system to new formats or products.
How does it work?
In a nutshell, the system is based on “machine learning”: the production operator “teaches” the system how to recognize a possible defect, adding new reference images as needed. However, it does not have to be a precisely defined defect: when analysing and processing the data, the system recognizes potential deviation from the norm.
What makes KSM Vision systems different from traditional solutions?
In order to compare the possibilities of modern, intelligent vision systems, we will give the example of a packaging and closure control system in a typical manufacturing process.
Traditional solutions are based on a set of several cameras capturing images of the product from multiple sides.
Meanwhile, the system we designed is based on a specially designed optical system. It enables comprehensive control of the entire tested object with a single camera. The mirror set patented by KSM Vision enables 360° circumferential inspection of the caps (patent PL No. 229618)
Compared to traditional systems, our vision system has far fewer electronic components, uses less space, is more accurate and can be easily integrated with existing production lines of our Customers.
As we mentioned above, our optical systems (based on neural networks) are also characterized by much greater precision and more options than traditional solutions: without manual setting of parameters or uploading a precisely defined pattern.
Our system is constantly “learning” using variable data that appear during the production process. It is more “reactive” to changes and deviations from a predefined pattern.
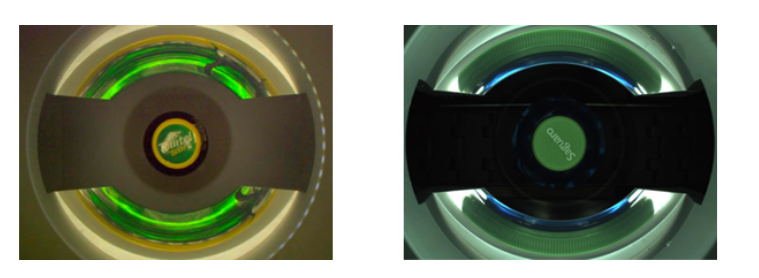
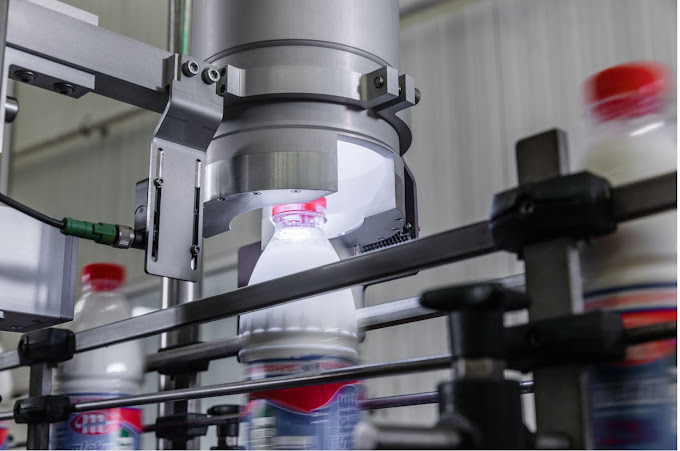
Use of automated quality control systems
Vision systems based on neural networks and the so-called machine learning are a perfect solution for the food, pharmaceutical, construction, textile industries, etc. – wherever a defect/deviation from the norm cannot be defined precisely.
Precision and quick detection of a defective product are extremely important as well – we are talking about fast, high-volume production lines. Failure to detect a possible production defect on time may result in producing a whole series of defective goods and huge production losses. Not to mention the risk of returns and sometimes the necessity to recall a whole series of products.
Of course, the application of our vision systems is not limited to the aforementioned industries. KSM Vision has developed a new version of the scanner that uses a colour camera and optimized the defect detection algorithm in order to increase the system performance. It forms the basis of the system for repairing boards used for the production of furniture. As a result, our solutions can be successfully used in production quality control in the construction industry or the wood industry, for example, for detecting and removing wood defects (cracks, resin pockets, dead or loose knots). Neural networks allow you to detect defects of heterogeneous products, i.e. products that are significantly different from each other, such as concrete or wood. Systems with classical image processing would need even dozens of parameters (manual optimization of parameters in a multi-dimensional space is virtually impossible).
Why should you choose intelligent modern quality control systems?
- flexibility and continuous “learning” of the system: additional detection of new defects that could not be foreseen during the system implementation,
- high sensitivity to production needs: as above – the operator can easily add new reference images and “re-train” the system
- higher capacity: the systems can be used on high-speed production lines working at a capacity of up to 70,000 pcs/h
- easy operation, accompanied by shorter training period for the operator (which is especially important when there is a fast employee turnover),
- the possibility to detect and classify defects,
- defect classification over a longer period of time may also help to optimize the technological process – e.g. a lot of defects of a certain type can be linked to a failure or suboptimal parameters of one of the production machines (learn more about the Inspectweb analysing and reporting application Inspectweb),
- easy integration of the system with existing production lines of the Customer,
- the systems can be installed in almost any industries.