Efficiency of x-ray in the quality inspection
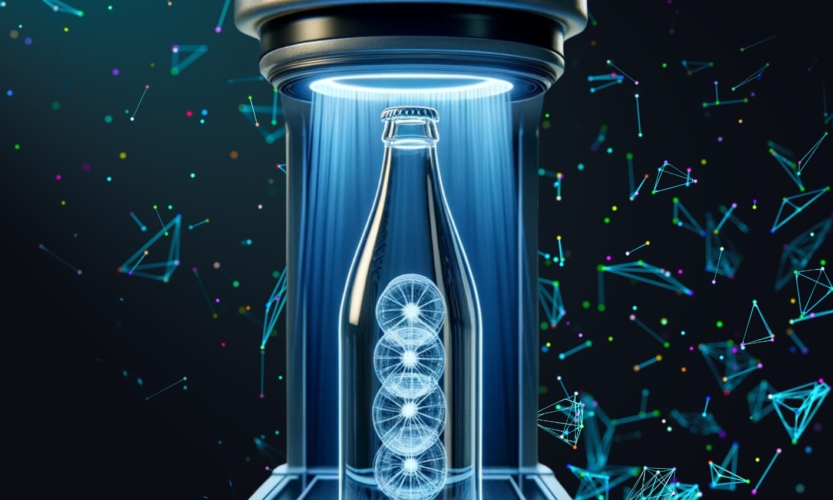
KSM Vision inspected the sealed food products using X-rays. Detecting impurities or foreign bodies in already packed products is not the easiest task. X-ray inspection came to the rescue.
Why X-Ray Control?
Quality control with X-Ray allows to test the quality of packed product in terms of presence of foreign bodies in it. These foreign bodies can be glass residues, metals, stones and much more. X-ray inspection will ensure that the product with undesirable content will never end up in the hands of the customer.
The quality requirements of food products also have to be noted. Due to the properties of comestible products, they are easy to destroy and require hygienic and sterile conditions for production. Quality control must be as minimal invasive and non-contact as possible.
In addition, X-Ray system is completely safe for the operator – the thickness of the inner sheath is calculated by the Radiological Protection Inspector, thanks to which radiation leaks are limited. Thanks to the cooperation with the Radiological Protection Inspector, the vision systems designed by KSM Vision ensure safe radiation level below 1 µSv/h and, as a result, are approved for operation by National Atomic Energy Agency.
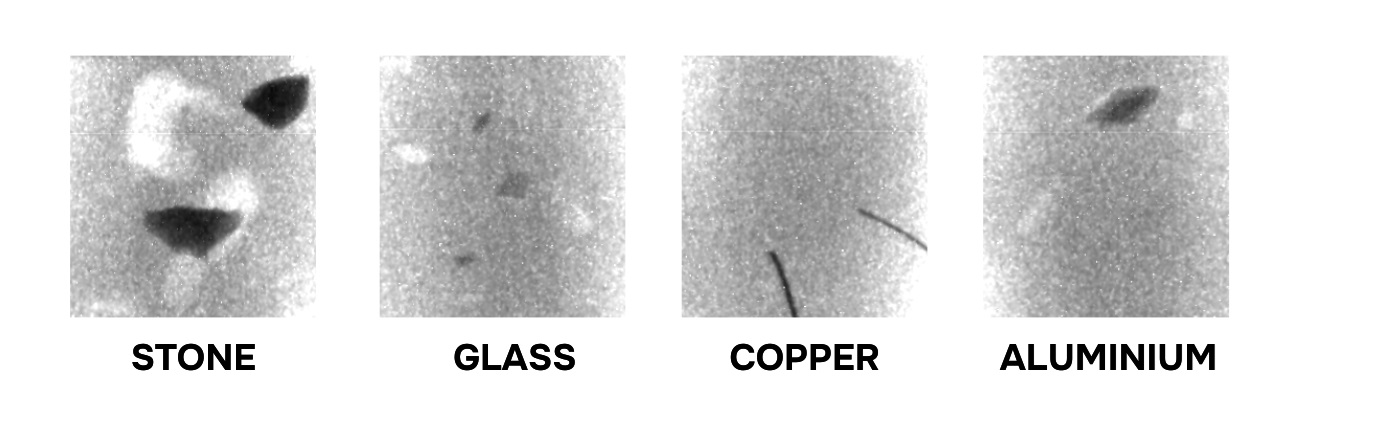
X-ray inspection challenges
Tests were carried out on 3 products: grated horseradish, tomato concentrate and an instant dish in a retortable pouch. The conducted tests allowed to assess the detectability of various types of foreign bodies in a closed package.
Each object, depending on its chemical composition and thickness, absorbs X-rays differently and, consequently, the parameters of the image acquisition system should be determined individually. In order to determine the level of detectability of foreign bodies in the tested products, two series of measurements were carried out on objects of known geometry. During the first series, the optimal system parameters were selected – the voltage and current intensity of the X-ray generator as well as the image acquisition parameters (gain, exposure time) were selected. During the second series, the detectability of foreign bodies was determined.
The next step in the inspection was to select the optimal photo recording parameters. In order to facilitate the tests, reference objects (defects) of known geometry and made of various materials were glued to the objects, they were:
- steel ball ∅2 mm,
- stone, dimensions of approx. 6 mm × 9 mm × 3 mm,
- 1.5 mm thick aluminum plate,
- electric cable ∅0.8 mm,
- 6 mm thick rubber cylinder,
- 10 mm thick plastic cylinder,
- glass balls ∅2.5 mm.
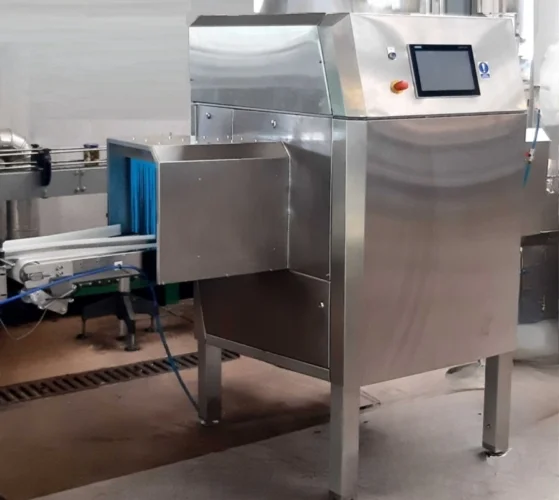
The conduct of the X-Ray tests
Measurements of the set of materials were made along with inclusions received from the manufacturer. Additionally, large pieces of cardboard and polystyrene were put in jars with tomato paste. For each sample, the measurement and qualitative assessment of the defect detection was performed. The qualitative assessment of the detection of defects is presented according to the adopted scale:
1 – invisible element, the discharge of which is similar to the tested sample. For example:
- plastic,
- gum,
- paper,
- organic waste.
2 – medium trace element, its detectability, title adjustment (thickness and shape) and location in the product. For example:
- aluminum balls less than 3 mm in diameter,,
- glass spheres less than 3/5 mm in diameter,,
- electric wire with a core diameter of less than 0.5 mm,
- stone balls with a diameter of less than 1 mm,
- wire sponge,
- cardboard,
- Styrofoam
3 – element clearly visible is detectable and facilitates the creation of progress. For example:
- steel balls with a diameter greater than 1 mm,
- glass balls with a diameter greater than 3 mm,
- aluminum balls with a diameter greater than 3 mm,
- an electric wire with a core diameter greater than 0.5 mm,
- stone balls with a diameter greater than 1 mm,
- chewing gum.
Conclusions from the use of RTG Quality Control
Just as the X-ray inspection was extremely effective in detecting foreign bodies, i.e. metals or stones, it was worse at dealing with materials, i.e. rubbers and organic waste (this is because they have X-ray absorption similar to the tested product, therefore they are they are virtually undetectable).
In the case of materials such as aluminum and glass, it has to be remembered that they must have the appropriate thickness – so that they are clearly visible in every part of the packaging. For example, glass is very visible in instant food pouches because their packaging is plastic, while in jars they are less visible (less contrast and smearing). Such defects can be detected using automatic image processing procedures.
Use of neural networks in the analysis of industrial radiography
It is worth pointing out the defect detection algorithms in the case of elements that are moderately visible for instant dishes. The visibility of these elements depends on their location in the product, because the intensity of the product itself in the photos is heterogeneous (granules of variable intensity). Some foreign objects will be clearly visible at the top of the sample, but invisible at the bottom. Classic image analysis will require numerous image transformations and setting dozens of dependent parameters (e.g. the permissible change of the calculated gradient). This type of approach can easily lead to analysis errors with small changes in the absorption of radiation by the product that result from its natural extraction method, e.g. a small change in product moisture. The answer to this problem is the use of neural networks for image analysis. In the learning process, the neural network automatically determines thousands of image features, and then, in the statistical analysis, it selects the parameters that best characterize foreign bodies.